"Why did they build it that way?"
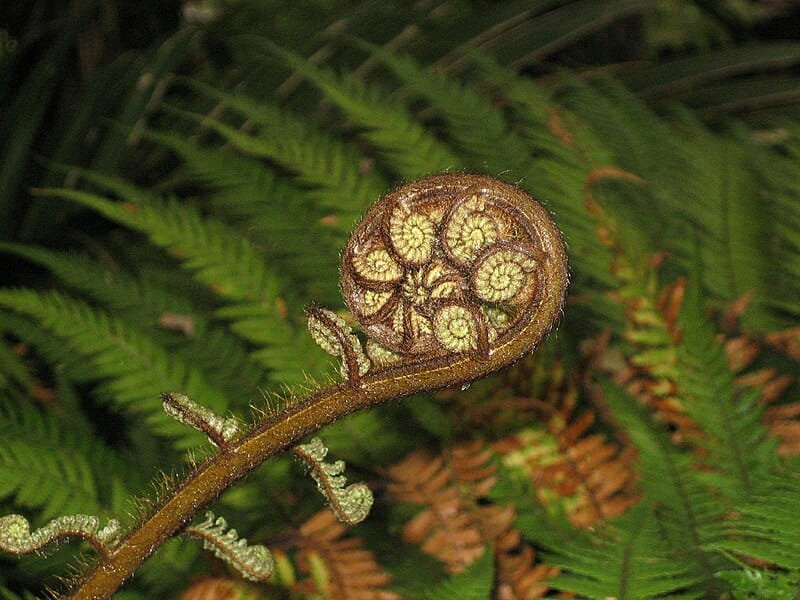
Thousands of scientific workflows are embedded in research papers, locked in PDFs, many behind paywalls.
Aggregators like Papers With Code have made these workflows more accessible by linking papers to their codebases. But one critical piece is still missing: the human insights that shaped these workflows.
Thanks for reading pluriverse work! Subscribe for free to receive new posts and support my work.
What reasoning, constraints, and trade-offs guided scientists in designing these tasks? Are these decisions unique to each field, or do common patterns emerge across disciplines? If we could systematically derive these insights and compare them, could we uncover a unified set of design principles for data use?
These principles exist in the abstract, but new computational approaches give us the opportunity to map the why behind scientific workflows, not just the how.
The Problem: Scientific Design Insights Are Scattered
Scientists document how they structure workflows, but not always why. Even when insights exist, they are fragmented across PDFs, paywalled journals, and discipline-specific silos. Without a way to compare these decisions across fields, we miss opportunities for shared principles and transdisciplinary innovation.
The Idea: Deriving Human Insights Computationally
What if we could systematically derive and compare the decision-making behind scientific workflows? By using computational methods like natural language processing, structured metadata, and large-scale analysis, we could surface patterns across disciplines, revealing the implicit design principles that guide research software development.
This wouldn't just save product managers and designers time during product discovery. It could also help scientists, developers, and data users design better workflows by learning from past thinking and decisions.
What Do You Think?
This idea comes from years of tracking down how others build scientific workflows to inform my own design strategies.
Tools like Dribbble and Behance cater to commercial, high-end aesthetics. But for designers working on scientific applications, literature reviews remains the primary real-world source of inspiration.
If this approach resonates with you, I would love your thoughts:
- What aspects of scientific workflows would be most valuable to analyze?
- How might this approach be applied in your field?
- What potential challenges or limitations should we consider?
As we incorporate more advanced technologies into research software, now is the time to build something that supports the people designing these tools.
Drop a comment or DM me. I am happy to connect with others thinking along these lines. Or, if you have already solved this problem, amazing! Please share.
Thanks for reading pluriverse work! Subscribe for free to receive new posts and support my work.